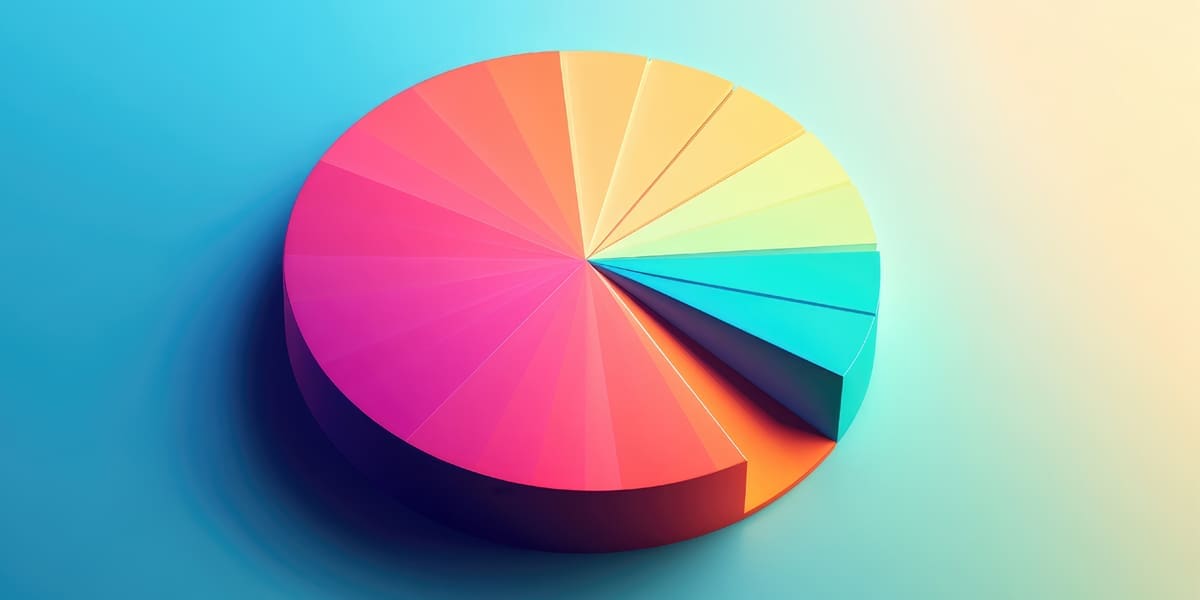
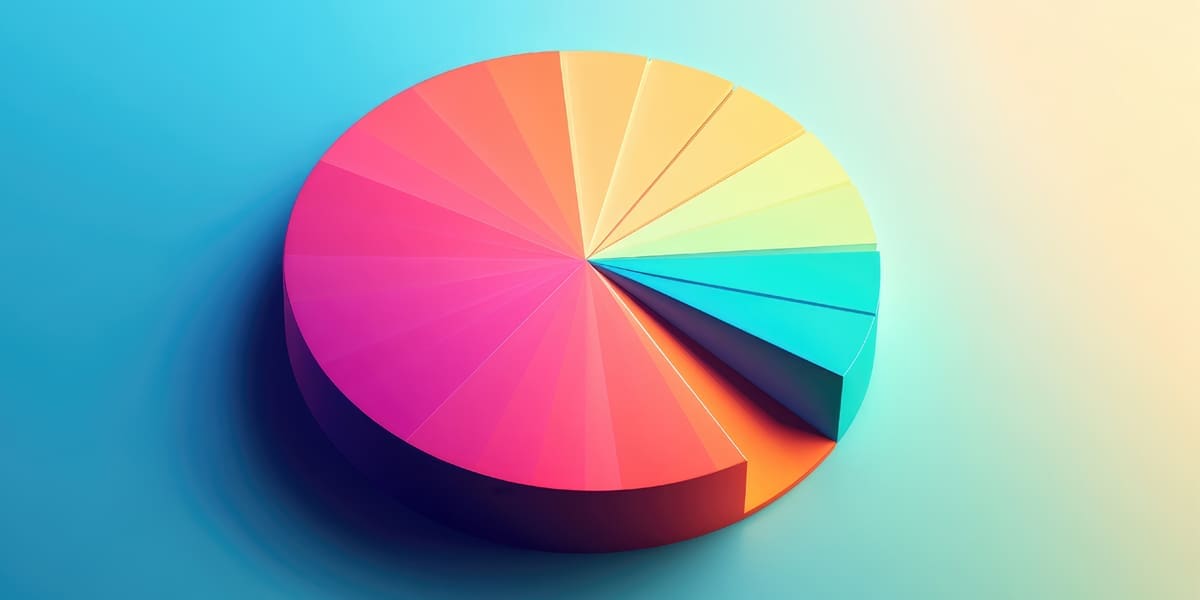
The Role of AI in Data Democratization
The Role of AI in Data Democratization
As organizations grapple with unprepared infrastructures and incomplete data strategies, the promise of data-driven decision-making remains out of reach for many. This data deluge also translates to siloed data silos, hindering the free flow of information across departments and hampering data-driven decision-making.
The lack of a unified data-to-AI approach leads to inconsistent data interpretation and hampers decision-making. Limited data accessibility and insufficient self-service options create bottlenecks that hinder innovation and agility. Many organizations struggle to unlock their data’s full potential as platforms prioritize cost or scale over comprehensive AI integration.
Traditional data management processes, burdened by technical debt, slow down AI data onboarding. Data silos fragment insights, complicating holistic analysis, while governance and classification challenges impede data security and access control.
This is where data democratization comes into play. This allows users across the organization to have access to and provide the ability to understand data, tackling the multifaceted landscape of challenges that collectively forms a formidable barrier to achieving true data democratization.
AI acts as the catalyst in this transformation, bridging the gap between complex data and actionable insights.
This blog post explores how AI is revolutionizing data democratization, empowering decision-makers at all levels to access and interpret crucial information. We will delve into the challenges of data silos and data overload, define data democratization, and showcase how AI technologies are driving this change.
What is Data Democratization?
Enterprises are now in the age of unprecedented data generation, with the global datasphere projected to grow to 175 zettabytes by 2025, a staggering increase from 33 zettabytes in 2018. Despite this abundance, many organizations struggle to translate this wealth of information into actionable insights.
A recent McKinsey survey revealed that 65% of organizations are now regularly utilizing generative AI, nearly double the adoption rate from just ten months prior. This significant increase highlights the critical role of data democratization in enabling broader access to advanced tools and insights, fostering more informed and data-driven decision-making across enterprises.
The challenge lies not just in collecting data, but in making it accessible and comprehensible to those who need it most – often non-technical decision-makers across various departments. This is where the concept of data democratization comes into play, promising to bridge the gap between raw data and actionable intelligence.
Data democratization is the process of making digital information accessible to the average non-technical user of information systems, without having to require the involvement of IT. It’s about breaking down data silos and empowering individuals across an organization to access, understand, and utilize data for informed decision-making.
Challenges in Data Management and AI Adoption
Despite the growing importance of data-driven decision making, many organizations face significant hurdles in their journey towards effective data management and AI implementation. For instance, a survey by NewVantage Partners has shown that only 30% of business organizations have a clearly defined data strategy.
This lack of readiness often stems from outdated or incompatible systems that struggle to handle the demands of a modern data and AI factory. While most modern data platforms or modern database estates meet scale or cost optimization plans, they fall short in providing a comprehensive capability to provide data to AI platforms.
Without a data strategy vision, organizations often find themselves overwhelmed by the sheer volume and complexity of their data assets. Even organizations with strategies focused on data analytics with data warehousing fall short of having an overarching data to AI strategy for predictive analytics and agentic AI usage.
Enterprises also face problems with a lack of data ops maturity, leading to a lack of trust in the data being collected. There is also a lot of opportunity cost lost by inefficient data management, hampering effective collaboration, automation, CI/CD, testing, monitoring and observability, especially when it comes to serving data to agentic AI platforms.
In addition, many businesses grapple with siloed data structures, making it challenging for employees across different departments to access and utilize crucial information effectively. Years of accumulating technical debt can lead to a complex, inefficient IT landscape that hinders progress towards data democratization and AI integration. This also leads to a lack of self service for accessibility and data prep for data analytics as well as broader AI applications stopping innovation in its tracks.
Despite these challenges, AI presents immense opportunities for organizations to revolutionize their approach to data management and accessibility.
AI’s Role in Data Democratization
Artificial Intelligence should be at the center of any enterprises’ data democratization. By using AI technologies such as large language models (LLMs), agentic AI, and automated analytics, organizations can make data analysis accessible to users without deep technical expertise.
According to a report by McKinsey, AI-powered data democratization tools can potentially unlock $9.5 trillion to $15.4 trillion in annual economic value globally. This staggering figure is just a marker of the immense potential of AI in changing how businesses interact with and derive value from their data.
AI can enable data democratization through many avenues, such as:
- Simplifying Complex Data: AI algorithms can process and summarize vast amounts of data, presenting insights in easily digestible formats.
- Natural Language Interfaces: NLP allows users to query databases using everyday language, eliminating the need for complex SQL knowledge.
- Automated Insights: AI can identify patterns and anomalies in data, proactively surfacing important findings to users.
- Predictive Analytics: Machine learning models can forecast trends and outcomes, empowering users to make data-driven decisions about the future.
Data Democratization: Benefits and Challenges
Let’s explore the key hurdles businesses face when their data foundation isn’t primed for AI initiatives.
Selecting a Unified Data Management Platform
Selecting the right unified data management platform is crucial.
Challenge: Many organizations find themselves grappling with a patchwork of niche platforms, each serving a specific purpose but failing to provide a cohesive data ecosystem. This fragmentation not only hampers data accessibility but also stalls advanced analytics and AI projects, preventing businesses from realizing the full potential of their data assets.
Business Impact: One of the most significant advantages of a unified data management platform is the dramatic reduction in time to access data for analysis and AI initiatives. By centralizing data from various sources, organizations can eliminate silos and streamline data retrieval processes. This efficiency boost allows data scientists and analysts to spend less time hunting for data and more time deriving valuable insights, accelerating innovation cycles and decision-making processes.
Managing a Decentralized Data Landscape
As data landscapes become increasingly decentralized, establishing cohesive data governance becomes a formidable challenge.
Challenge: Without a unified approach, organizations struggle to maintain data quality, ensure compliance, and foster trust in their AI-driven insights. This lack of governance can lead to inconsistent results and erode confidence in AI initiatives.
Business Impact: AI-driven data management platforms can automate many aspects of data governance, including data classification, access control, and audit trails. This not only ensures adherence to regulatory requirements but also builds trust with customers and stakeholders by demonstrating a commitment to responsible data handling.
The Self-Service Analytics Gap
Limited self-service analytics capabilities can create bottlenecks in the AI development process.
Challenge: When data scientists and business users lack easy access to the data they need, it not only slows down innovation but also hinders the organization’s ability to respond quickly to market changes and customer needs.
Business Impact: A unified platform uses automation to simplify manual, time-consuming tasks, reducing the need for extensive hand-coding and manual data preparation. This shift increases efficiency, reduces the barrier for entry, and allows data teams to focus on higher-value activities that drive innovation and business growth.
Bridging AI and Business Intelligence
Ensuring that AI technical strategies enhance rather than replace existing business intelligence functionalities is a delicate balance. Organizations must find ways to integrate AI seamlessly into their current analytics ecosystem, augmenting human decision-making rather than creating isolated AI silos.
Business Impact: By integrating AI capabilities into the data management process, organizations can create an AI-powered data supply chain. This approach enhances data quality, automates data cataloging, and facilitates advanced analytics. The result is a more agile and responsive data ecosystem that can adapt to changing business needs and support diverse AI use cases across the organization.
The automation of data curation, preparation, and integration, helps AI-powered data supply chains break down traditional barriers to data accessibility.
Creating an AI-Powered Data Supply Chain with 66degrees
To address these challenges efficiently, organizations can benefit from partnering with innovative solution providers. 66degrees, we design and deploy unified data/AI platforms that drive innovation. Our approach accelerates AI-driven BI development, enhances data reliability, and simplifies ecosystems for easier team access. With orchestrated data pipelines, automated processes, and intelligent data preparation, we ensure seamless integration and scalability across your organization.
Our Metadata-Driven Design, Data Quality Framework, and Data Catalog Implementation guarantee data integrity and improve accessibility, empowering your teams to drive operational excellence. Our Data Mesh Architecture connects data management strategies with BI/AI goals, enabling self-service for business and AI teams, and offering flexible data access models (decentralized or centralized) with strong security. Our AI-powered Data Supply Chain streamlines data collection, cleaning, and analysis, integrates data into AI solutions, and provides contextual enrichment and clear data lineage.
At 66degrees, we empower organizations to make the right choices when it comes to cloud modernization and setting the stage for AI integration. Our strategic Google Cloud consulting services help align your technology needs with your business objectives, ensuring a robust, future-proof AI infrastructure. By making use of our deep expertise in cloud, data and AI engineering, we guide you through every step of your transformation journey. Connect with us to learn how we can help.