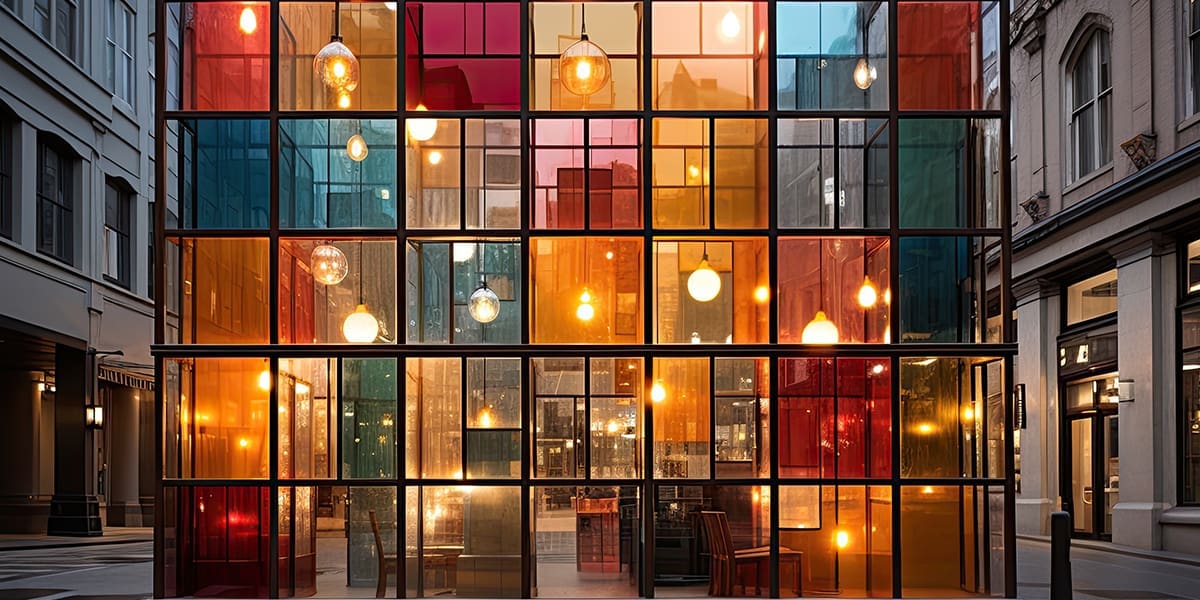
Personalized Shopping Experiences: AI’s Role in Curating Customer Data for Targeted Marketing
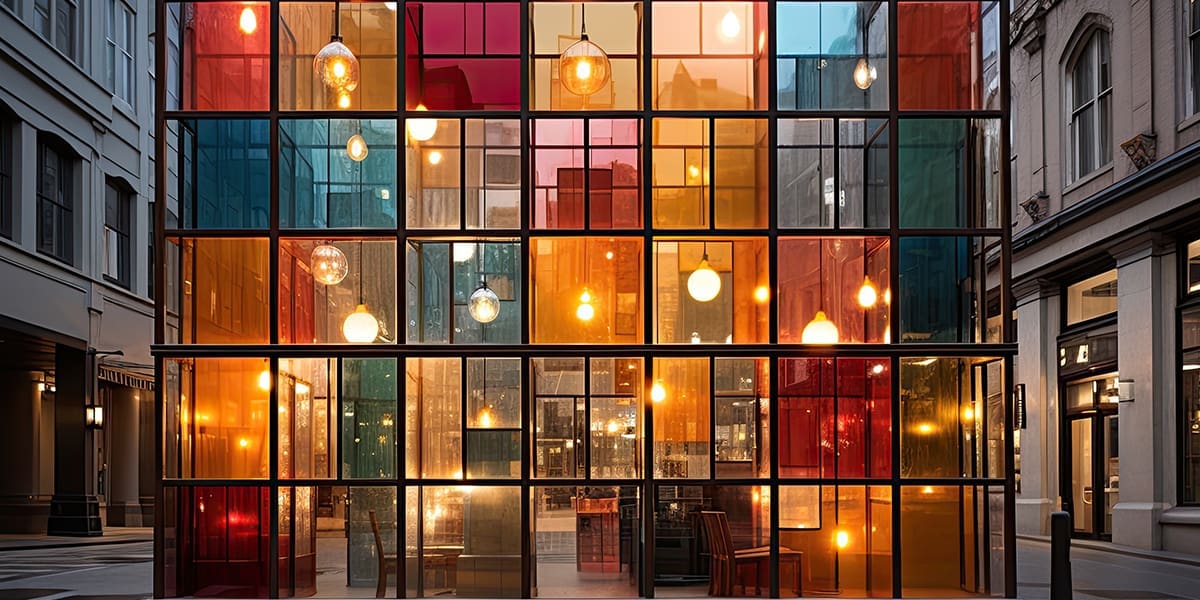
Personalized Shopping Experiences: AI’s Role in Curating Customer Data for Targeted Marketing
Have you ever wondered how some digital platforms seem to understand your needs almost before you do? Imagine exploring an online resource for your next big project, only to find that the site instantly presents you with tailored insights, case studies, and recommendations that resonate with your unique challenges. This is not serendipity—it’s the result of AI- driven personalization.
The urgency for tailored experiences is clear. Reflecting this trend, 51% of CMOs and marketing leaders plan to boost investments in digital shelf content and personalized commerce touchpoints over the next 12 months.
Yet personalization remains a puzzle for businesses.Why so? – Organizations gather vast amounts of data, but only 30% have a clearly defined strategy to transform that data into actionable insights. Data silos, and inefficient ETL processes hinder seamless, real-time interactions. In response, many turn to AI as a transformative solution that curates, organizes, and activates customer data for precision-targeted engagement—delivering dynamic experiences that traditional approaches simply cannot match.
But are we AI- ready? Despite AI’s promise, many organizations find themselves unprepared for its demands. Integration complexities—such as unprepared enterprise infrastructures, fragmented data-to-AI strategies, and mounting technical debt—impede progress. Bridging data silos, ensuring data accessibility, and building scalable systems that foster continuous innovation remain significant challenges.To overcome these challenges, businesses need unified data platforms that enable real-time personalization, streamline data preparation, and enhance AI-driven governance.
In this blog, we explore how to navigate these challenges and transform data complexity into a strategic asset that drives measurable business outcomes through real-time personalization.
What is AI in Personalization?
AI driven personalization moves beyond static segmentation, dynamically adjusting recommendations and content to meet evolving customer needs. This shift is evident, with 80% of customer service and support organizations expected to adopt generative AI technology to enhance agent productivity and customer experience (CX). Moreover, AI enables cross-departmental collaboration by providing consistent, data-driven insights that align marketing, sales, and service strategies. This approach not only enhances customer experiences but also supports scalable, future-ready personalization initiatives that drive long-term business growth.
Why AI-Driven Personalization Matters
Driving Cost Efficiency: AI automation minimizes manual data preparation and processing, reducing operational overhead and accelerating insights. This shift optimizes resource allocation and lowers long-term data management costs.
Enhancing Data Value: AI transforms raw data into strategic assets by ensuring it is contextually enriched, accessible, and ready for real-time insights. This unlocks higher value from existing data infrastructures.
Scaling Personalization Efforts: AI enables organizations to expand personalization initiatives without a proportional increase in costs, ensuring scalable growth while maintaining consistent quality.
AI as the Solution: Transforming Data into Personalization
AI empowers businesses to overcome data fragmentation and deliver real-time, hyper-personalized experiences through several key strategies:
1. Unified Data Platforms for Real-Time Personalization
Fragmented data systems and technical debt are major barriers to seamless integration and personalization. Unified data platforms overcome these obstacles by integrating structured and unstructured data, creating comprehensive profiles for dynamic, cross-channel personalization.
By breaking down silos and centralizing information, organizations can ensure cohesive customer experiences across digital and physical touchpoints while enabling scalability for real-time personalization.
2. Streamlining Data Preparation with DataOps
Traditional data preparation is often slow, manual, and fragmented, contributing to inefficiencies and delays. Embedding DataOps practices helps automate data collection, enrichment, and preparation. This ensures data is clean, structured, and ready for analysis, reducing manual overhead and accelerating insights.
DataOps also promotes better collaboration, testing, monitoring, and observability across data processes, addressing challenges related to data governance and accuracy. This approach empowers teams with self-service access, minimizing bottlenecks and enabling faster, more effective personalization strategies.
3. Forming an AI-Powered Data Supply Chain To overcome challenges related to decentralized data and limited accessibility, businesses can develop an AI-powered data supply chain. This approach decentralizes data, contextually enriches it, and automates quality checks and delivery processes.
Key strategies include:
- Dynamic Recommendations: Leveraging behavioral insights to offer timely, relevant product suggestions.
- Targeted Outreach: Utilizing historical engagement data to tailor emails, ads, and outreach efforts.
- Conversational Support: Implementing predictive assistance through chatbots or customer service platforms to enhance customer satisfaction.
By activating these processes, businesses can deliver more personalized and intuitive customer journeys while reducing the impact of fragmented data landscapes.
4. Strengthening Governance and Trust Data governance and security are essential to building consumer trust in personalization strategies. Automated governance processes ensure compliance with regulations like GDPR and CCPA while maintaining ethical data practices.Robust security frameworks protect sensitive data, mitigating the risk of breaches.
Additionally, automated classification and inventory processes help manage data more effectively, ensuring it is consistently monitored and securely handled. This fosters trust and confidence in data-driven personalization efforts.
Implementation Strategies: Turning Vision into Reality
Successfully implementing AI-powered personalization requires a strategic approach aligned with modern data architectures and practices:
- Establish Modern Data/AI Platforms: Build infrastructures that integrate a Data Mesh approach to reduce ELT overhead and minimize data duplication. Enable federated querying across Google Managed DBs, Business Domains, and Cloud Storage for efficient, scalable analysis.
- Develop DataOps and Governance Programs: Implement a strong Data Ops control plan to ensure data security, governance, and orchestration. This will enhance data quality while ensuring regulatory compliance and operational efficiency.
- Adopt a Data Mesh Architecture: Decentralize data ownership through Data Contracts that enforce downstream dependencies, ensuring data integrity and streamlined analysis. Utilize Data Agents to facilitate secure, efficient data movement across the mesh for analysis, predictions, and AI consumption.
- Enable Real-Time and Legacy Data Integration: Leverage CDC Replication to bring legacy Data Center application data into the lake. Support Real-Time Ingestion to process event data as it occurs, enabling immediate model calls or storage for later analysis.
- Optimize Data Access and Structure: Establish a Data Lake as an access layer across all data assets, organized by business domains. This supports unstructured, semi-structured, and structured datasets, providing a comprehensive view for analytics and AI models.
- Start Small, Scale Fast: Focus on high-impact, immediate-value use cases. Leverage the scalability of the mesh and data lake to expand incrementally while maintaining data consistency and performance.
- Balance Automation with Human Touch: While AI insights drive efficiency, ensure human creativity augments personalization efforts, delivering authentic and engaging experiences.
By aligning with these strategies, organizations can transform data into actionable insights, enhancing personalization and driving business growth.
Key Trends In AI Driven Personalization
As customer expectations for seamless, relevant experiences grow, AI-driven personalization must evolve to meet these demands. Here are the key trends shaping the future:
- Hyper-Personalization at Scale:As customer expectations rise, businesses that deliver meaningful, tailored experiences will gain a competitive edge—with $2 trillion in revenue expected to shift to those excelling in personalization over the next five years. This makes personalized engagement a key driver for future growth.
- AI Agents for Intelligent Engagement: AI agents such as customer service chatbots are transforming engagement by enabling dynamic, real-time interactions. These agents also simplify internal processes by supporting intuitive data exploration and preparation.
- Omnichannel Personalization: AI ensures consistent personalization across all touchpoints—whether digital, in-store, or service-based—enhancing cohesive customer experiences.
- Secure AI Infrastructure: As data privacy concerns grow, organizations are adopting AI frameworks that automate governance and compliance while strengthening security. This approach is vital, with nearly 60% of global respondents identifying improved threat detection as the top benefit of integrating AI into cybersecurity operations, reinforcing AI’s role in safeguarding data.
These trends underscore the critical role of AI in shaping personalized experiences that drive business growth.To explore how AI is transforming customer experiences and driving business innovation, download our eBook— AI Business Trends 2025.
Conclusion: Leading the Future of Personalized Engagement
AI is reshaping how businesses approach personalization. By transforming vast datasets into actionable insights, AI enables real-time, hyper-personalized experiences that resonate with modern consumers. However, the journey requires more than technology. It demands strategic investment, robust infrastructure, and a relentless focus on data quality and governance.
Advancing AI-driven personalization requires more than technology—it requires a strategic, data-first approach.That’s why, at 66degrees, we build AI-ready platforms that align with business goals and scale for future growth, ensuring deeper engagement and competitive advantage.We prioritize robust data infrastructures and cross-departmental collaboration. By leveraging Data Mesh for efficient data management, enforcing data integrity through contracts, and enabling real-time and legacy data integration, we ensure AI outputs are relevant and reliable.Our approach empowers organizations to scale AI-driven personalization effectively.
For instance, we helped a financial solutions provider overcome data fragmentation by centralizing their data, implementing a self-service dashboard, and leveraging machine learning for deeper insights.This approach not only improved decision-making but also enhanced customer engagement and business growth.To know more,explore the full case study here.