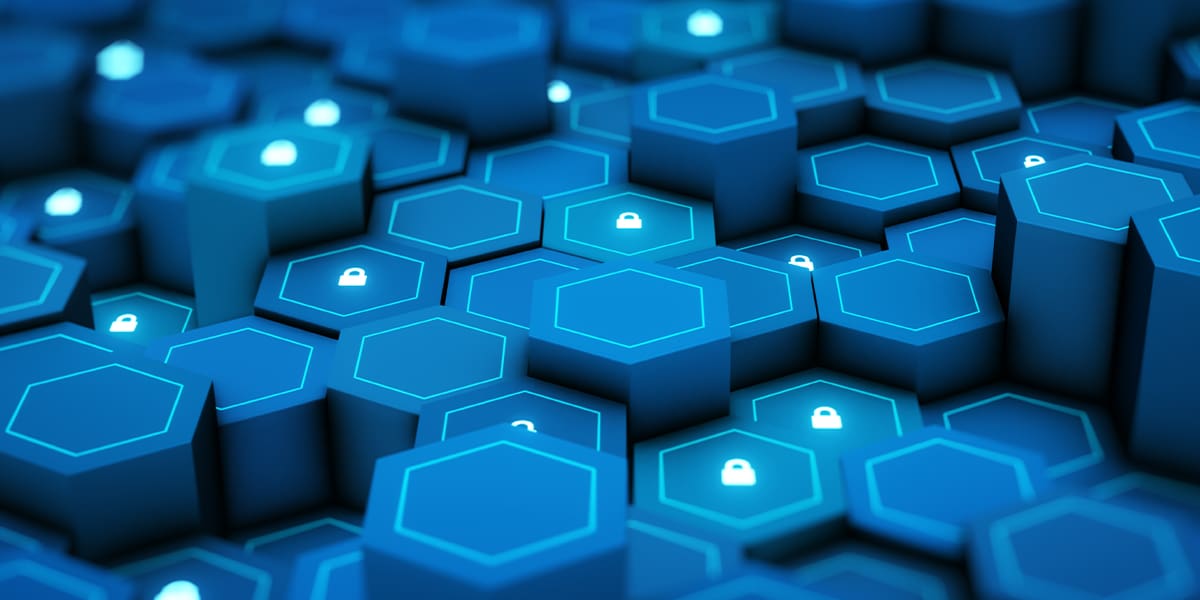
Fraud Detection and Prevention: AI-Enabled Applications and a Secure Cloud Foundation
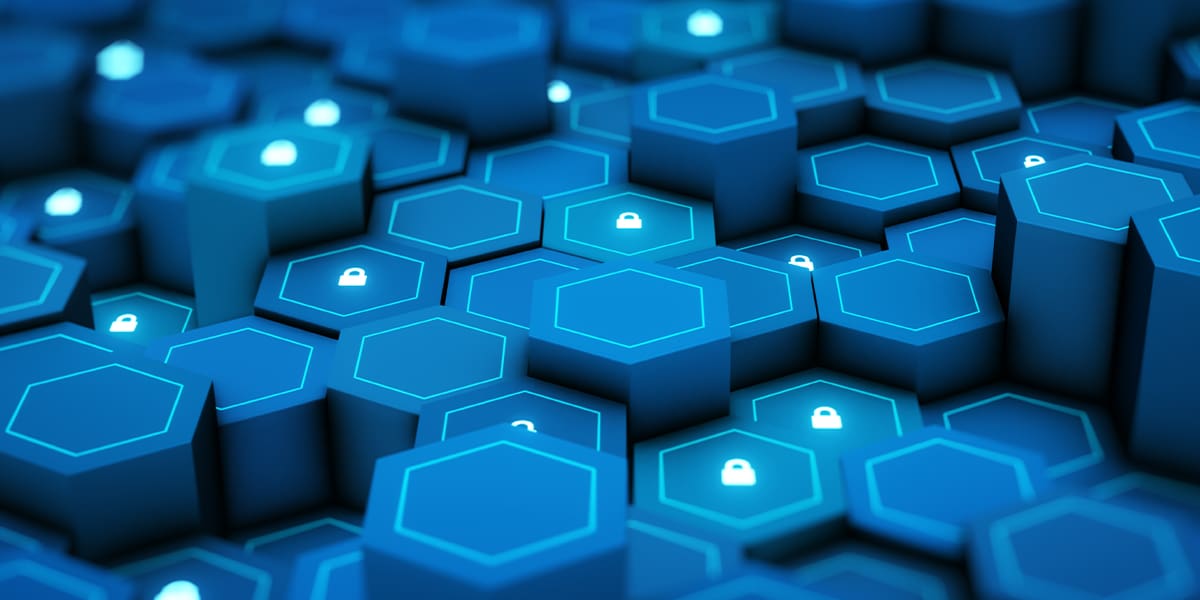
Fraud Detection and Prevention: AI-Enabled Applications and a Secure Cloud Foundation
The financial impact of fraud continues to escalate at an alarming rate. According to recent research, organizations lost an estimated $3.1 billion to fraud in 2023, with a median loss of $117,000 per case. Besides, the Association of Certified Fraud Examiners (ACFE) reports that businesses lose approximately 5% of their revenue to fraud annually.
What’s more troubling is that a typical fraud case persists for about 12 months before detection—making recovery virtually impossible.
A secure cloud infrastructure, strengthened by AI,is essential to mitigating these risks. However, many organizations remain hesitant to embrace cloud solutions due to security concerns, a lack of cloud expertise, and the complexities of implementation.
Security remains a top priority,yet without a clear understanding of cloud security frameworks, organizations struggle to trust that their data and applications will be safeguarded against breaches and unauthorized access.As per KPMG, revealed that 78% of professionals are concerned about their ability to keep pace with technological advancements, while 80% believe that their organization’s risk-averse leadership causes them to lag behind competitors in embracing new technologies.
At the same time, organizations are under increasing pressure to accelerate application development and deployment. Any cloud solution must not only enhance security but also streamline operations without disrupting workflows or innovation.
In this article, we’ll explore how AI is transforming fraud detection and prevention, examine the strategic implementation of these technologies, and discuss the cloud security foundation necessary to support these powerful tools
Understanding AI-Powered Fraud Detection Fundamentals
As digital transactions increase, so do fraud attempts, creating a growing challenge for businesses and consumers alike. Between 2022 and 2023, consumer reports for fraud, identity theft, and scams surged from 5.15 million to 5.4 million, underscoring the escalating threat landscape. Among these, identity theft remained the most commonly reported complaint, highlighting how fraudsters are increasingly exploiting stolen personal data for financial gain.
To combat these evolving threats, AI-powered fraud detection provides a more adaptive and proactive approach than traditional methods. By analyzing vast datasets in real-time, AI can detect subtle anomalies and patterns that signal fraudulent activity across various attack types, including:
- Transaction fraud: Unauthorized purchases, wire transfers, and payments made with stolen credentials or account information.
- Identity theft: The misuse of personal information to open accounts, secure loans, or conduct transactions under someone else’s name.
- Account takeover: When fraudsters gain access to legitimate user accounts through credential theft, social engineering, or security vulnerabilities.
- Application fraud: Submission of falsified information to obtain credit, loans, or other financial services.
- Synthetic identity fraud: Creating fictitious identities by combining real and fabricated personal information.
The integration of advanced machine learning algorithms significantly enhances AI capabilities in fraud detection. These algorithms include:
- Supervised learning: Models trained on labeled datasets that include known fraudulent and legitimate transactions.
- Unsupervised learning: Algorithms that identify patterns and anomalies without prior examples, detecting previously unknown fraud schemes.
- Deep learning: Neural networks that analyze complex patterns across multiple data dimensions, mimicking human cognitive processes.
- Reinforcement learning: Systems that improve through feedback loops, continuously adapting to new fraud tactics.
With these components and algorithms, AI-powered fraud detection systems can analyze vast amounts of data in real-time, identifying subtle patterns and connections that would be impossible for humans or traditional rule-based systems to detect.
Strategic AI Implementation for Fraud Prevention
Effective fraud prevention requires strategic implementation of AI technologies focused on several key capabilities:
- Pattern Recognition & Anomaly Detection
AI models establish behavioral baselines for users, transactions, and accounts, flagging deviations that may indicate fraud. Real-time detection enhances security while supporting rapid application development—critical for organizations balancing agility with risk management. - Behavioral Analytics & User Profiling
By analyzing device usage, transaction habits, and interaction patterns, AI enhances fraud detection while ensuring compliance with security protocols. Ethical considerations, including transparent data policies and bias mitigation, are essential for trust and adoption. - Advanced Machine Learning Models
A combination of supervised, unsupervised, and hybrid models enables organizations to detect known fraud patterns while identifying emerging threats. Continuous learning enhances accuracy, reducing false positives and strengthening proactive risk mitigation.
These advanced models can adapt to evolving fraud tactics, continuously learning from new data and improving their detection capabilities over time. As fraud techniques become more sophisticated, the self-learning nature of these systems provides a critical advantage in the ongoing battle against financial crime.
Building a Secure Cloud Foundation for AI Fraud Detection
To effectively implement AI-powered fraud detection, organizations must invest in a secure, scalable, and compliant cloud environment. This investment is crucial as the shift to cloud computing introduces new vulnerabilities. According to Gartner, cloud security spending is projected to grow by 24%, marking it as the fastest-growing segment in the global security and risk management market.
This trend underscores the importance of establishing robust security frameworks, automated governance, and reliable access controls to protect sensitive financial data and facilitate seamless AI adoption.
- Automated Security with Infrastructure as Code (IaC): IaC helps create secure, consistent cloud environments by automating security policies, tracking changes, and enabling quick recovery from breaches or failures.This reduces the risk of misconfigurations and ensures compliance.
- Strong Platform Security: AI fraud detection platforms must have data encryption, microservices architecture, and continuous security monitoring to prevent breaches and maintain system integrity.
- Compliance & Governance: Organizations must adhere to GDPR, PCI DSS, and AI-specific regulations by using built-in compliance reporting, audit logging, and AI model transparency measures.
- Robust Access Control: Implementing multi-factor authentication (MFA), role-based access (RBAC), and just-in-time access (JIT) ensures only authorized users interact with sensitive fraud detection systems, minimizing security risks.
By integrating these security measures, organizations can confidently adopt AI-driven fraud detection while maintaining compliance, reducing risk, and accelerating deployment. A well-structured cloud foundation not only strengthens fraud prevention but also simplifies adoption, ensuring efficiency and trust in AI systems.
To delve deeper into building a robust cloud infrastructure that supports secure and efficient AI adoption, explore our latest ebook, “Building the Foundation for AI Success.” This comprehensive guide offers insights into creating a secure, compliant, and scalable cloud environment, empowering your organization to harness the full potential of AI-driven fraud detection.
Real-Time Threat Detection and Response
The effectiveness of AI-powered fraud prevention depends on its ability to detect and respond to threats in real-time. This capability requires several integrated components:
Automated Monitoring Systems
Continuous, automated monitoring forms the foundation of real-time threat detection. These systems:
- Analyze transaction data streams as they flow through financial systems
- Apply multiple AI models simultaneously to evaluate risk from different perspectives
- Compare current activities against historical patterns and known fraud indicators
- Adjust sensitivity dynamically based on risk levels and emerging threats
Unlike traditional batch processing methods that might review transactions hours or days after they occur, real-time monitoring evaluates activities as they happen, enabling immediate intervention before funds leave the financial system.
Instant Alert Mechanisms
When suspicious activities are detected, instant alert mechanisms ensure that appropriate stakeholders are notified immediately. These mechanisms include:
- Risk-based alerting: Alerts are prioritized based on the likelihood and potential impact of fraud, ensuring that high-risk situations receive immediate attention.
- Multi-channel notifications: Alerts are delivered through multiple channels (dashboards, email, SMS, mobile apps) to ensure timely awareness.
- Contextual information: Alerts include relevant context, such as customer history, similar past incidents, and recommended actions.
- User verification workflows: In appropriate cases, customers are directly contacted to verify suspicious transactions before they’re processed.
These alert mechanisms bridge the gap between detection and response, ensuring that potential fraud is addressed promptly.
Response Automation
Automated response capabilities enable immediate action when fraud is detected, significantly reducing potential losses. These capabilities include:
- Transaction blocking: Automatically preventing suspicious transactions from being completed.
- Account restrictions: Temporarily limiting account functionality until the situation is resolved.
- Step-up authentication: Requiring additional verification for high-risk activities.
- Adaptive friction: Dynamically adjusting security measures based on risk level, adding friction only when necessary.
Response automation operates on predefined rules and risk thresholds, allowing organizations to take immediate action while maintaining appropriate human oversight for complex cases.
Integration with Existing Security Tools
AI-powered fraud detection systems must integrate seamlessly with existing security infrastructure to provide comprehensive protection. Key integration points include:
- Security information and event management (SIEM) systems that aggregate and correlate security data from multiple sources.
- Identity and access management (IAM) platforms that control user authentication and authorization.
- Threat intelligence feeds that provide up-to-date information on emerging fraud tactics and known threat actors.
- Case management systems that track investigation workflows and outcomes.
These integrations create a unified security ecosystem, allowing fraud detection systems to leverage broader security contexts and coordinate responses across multiple defensive layers.
66degrees: Driving Secure & Scalable AI Fraud Detection
One way to overcome these challenges is by leveraging expert guidance and strategic frameworks. At 66degrees, we ensure seamless AI implementation, regulatory compliance, and organizational readiness. Our secure cloud infrastructure, automated compliance frameworks, and streamlined approval processes mitigate security risks while ensuring adherence to GDPR, PCI DSS, and AI governance standards.
For example, we modernized a leading enterprise’s data infrastructure with Snowflake, Matillion ETL, and MicroStrategy on Google Cloud, enabling real-time analytics and scalability. The result? Faster reporting, enhanced fraud detection, and a future-ready platform. Explore the full success story to see how our solutions can empower your business.
At 66degrees, we empower organizations to make the right choices when it comes to cloud modernization and setting the stage for AI integration. Our strategic Google Cloud consulting services help align your technology needs with your business objectives, ensuring a robust, future-proof AI infrastructure. By making use of our deep expertise in cloud, data and AI engineering, we guide you through every step of your transformation journey. Connect with us to learn how we can help.