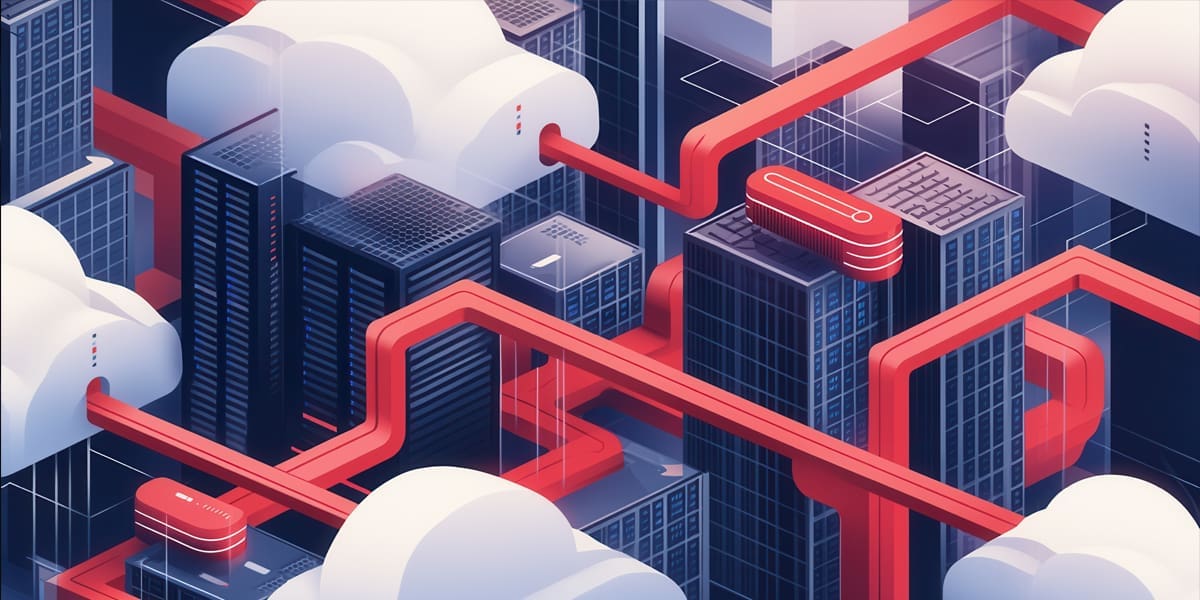
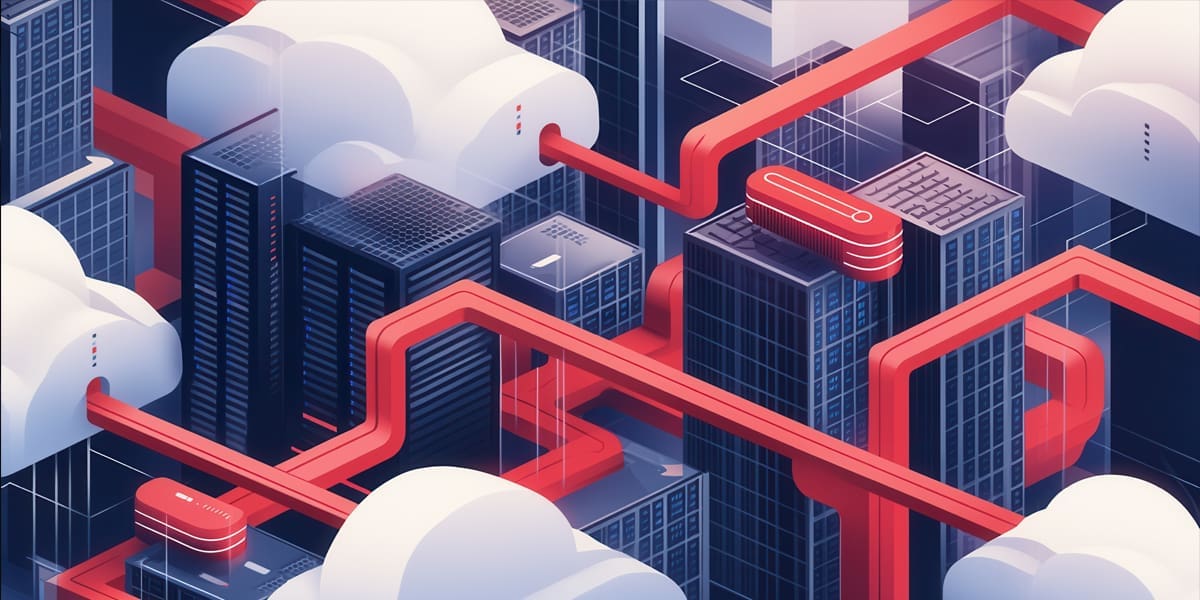
Data Modernization with Google Cloud: The Foundation of Enterprise AI
Data Modernization with Google Cloud: The Foundation of Enterprise AI
If your organization is struggling to launch successful AI initiatives, outdated data silos and inefficient data pipelines may be the root cause. When you don’t have a well-defined enterprise transformation strategy, these data infrastructure challenges can seem insurmountable.
The answer? A modern data foundation for AI.
Data modernization is revolutionizing how enterprises make use of AI, with improved data quality, scalability and AI-driven data insights. According to market research by IDC, siloed data can cost organizations upto 30% in annual revenue, showing how modernizing data infrastructure has become beyond crucial.
This blog takes a look at how Google Cloud’s data modernization solutions empower businesses to break down barriers, enhance analytics capabilities, and drive AI-powered innovation. We’ll look into the key strategies for successful implementation, the role of cloud-native technologies, and how a human-centric approach ensures that data modernization aligns with your organization’s unique needs and goals. Let’s dive in.
Understanding Data Modernization
Data modernization is the process of transitioning from legacy databases and infrastructure to modern, cloud-based systems that can handle the demands of modern days data-intensive applications. Moving data to the cloud is only one aspect of the problem; another is rethinking how data is collected, stored, processed, and utilized across the entire organization.
Key components of a modern data infrastructure include:
- Cloud-native architecture
- Real-time data processing capabilities
- Advanced analytics and machine learning tools
- Robust data governance and security measures
- Scalable and flexible data storage solutions
The importance of data modernization in preparing for enterprise AI cannot be overstated. Modern data platforms provide the agility, scalability, and processing power needed to train and deploy sophisticated AI models. They enable organizations to use the full potential of their data assets, driving innovation and competitive advantage.
However, the process of modernization can often be met with challenges.
Common hurdles in legacy data systems include:
- Data silos and fragmentation
- Inconsistent data quality and formats
- Limited scalability and performance issues
- Inadequate security and compliance measures
- Difficulty integrating with modern tools and applications
66degrees utilises cloud-native architecture to dismantle data silos, enabling seamless integration across various systems and limiting performance issues. They have also successfully aided Dematic by moving the legacy applications to Google Cloud Platform (GCP). By doing so, 66degrees facilitated a unified data ecosystem, allowing for improved data accessibility and collaboration across departments.
The Role of Google Cloud in Data Modernization
Google Cloud is revolutionary when it comes to data modernization, offering a comprehensive suite of tools and services designed to transform enterprise data infrastructure. With its unparalleled expertise in managing massive datasets and pioneering AI technologies, Google Cloud provides a robust foundation for organizations looking to modernize their data ecosystems.
Key features of Google Cloud’s data modernization capabilities include:
- Data Warehousing with BigQuery: A serverless, highly scalable, and cost-effective data warehousing solution for analyzing massive datasets with high performance.
- Data Lakes and Data Storage: Offers a range of storage options, including Cloud Storage for object storage, Cloud SQL for relational databases, and Cloud Spanner for globally distributed databases, providing flexibility for various data needs.
- Data Integration and Pipelines: Provides tools like Dataflow for building and managing data pipelines, enabling efficient data ingestion, transformation, and movement across different data sources.
- Data Governance and Security: Offers robust security features such as encryption, access control, and data loss prevention to ensure data privacy and compliance.
- AI and Machine Learning Integration: Seamlessly integrates with Google Cloud’s AI/ML services, allowing businesses to easily build and deploy machine learning models on their data.
- Open Source Support: Supports a wide range of open-source technologies, providing flexibility and choice for businesses.
- Strong Partnerships: Collaborates with a vast ecosystem of partners, offering a wide range of solutions and expertise to support data modernization initiatives.
Google Cloud’s data modernization solutions integrate smoothly with existing enterprise systems, allowing for a phased approach to migration. This flexibility enables organizations to modernize at their own pace, minimizing disruption to ongoing operations while gradually unlocking the benefits of a cloud-native data infrastructure.
Integrating AI into IT Infrastructure
The intersection of AI and IT operations represents a new aspect in enterprise technology. As organizations modernize their data infrastructure, they unlock opportunities to embed AI and machine learning capabilities directly into their IT operations.
Development bottlenecks may be avoided, manual integration time can be reduced, and overall system productivity can be increased when hardware and software are combined to create a cohesive, user-friendly, safe, and dependable computer system. Although hardware offers the raw potential, a strong ecosystem of tools, frameworks, and resources is necessary to fully realize it. These include:
- Extensive support for well-known machine learning frameworks
- Deep integration with tools (typically also driven by gen AI)
- Compute resource management’s inherent flexibility
- Open software for orchestration of AI workload
Few key strategies to consider when implementing AI with modernized data frameworks include automating data pipelines to streamline data ingestion, cleansing, and preparation processes.
Furthermore, predictive maintenance can be leveraged using machine learning models to anticipate and prevent IT infrastructure issues before they occur. Intelligent resource allocation, facilitated by AI-driven systems, optimizes cloud resource usage based on real-time demand and historical patterns.
The benefits of AI-driven IT infrastructure are substantial:
- Improved operational efficiency through automation of routine tasks
- Enhanced decision-making capabilities with real-time insights
- Increased system reliability and uptime
- Optimized resource utilization and cost management
- Proactive problem resolution and reduced mean time to repair (MTTR)
For better clarity on how to build a robust AI foundation for your business, check out this e-book on Building the Foundation for AI Success
Benefits of Modern Data Lakes for AI and Machine Learning
Modern data lakes are a vital component of AI-ready architecture, providing a centralized repository for storing vast amounts of structured and unstructured data. Unlike traditional data warehouses, data lakes offer the flexibility and scalability needed to support diverse AI and machine learning initiatives.
Key benefits of modern data lakes include:
- Centralized Data Storage: Modern data lakes provide a central repository for all types of data, including structured, semi-structured, and unstructured data, eliminating data silos and improving data accessibility.
- Improved Data Agility: By storing data in its native format, modern data lakes enable faster data access and analysis, allowing businesses to respond quickly to changing market conditions and gain a competitive edge.
- Scalability: Data lakes can grow seamlessly to accommodate increasing data volumes without performance degradation.
- Cost-Effectiveness: By making use of cloud storage, data lakes offer a more economical solution for storing large datasets compared to on-premises alternatives.
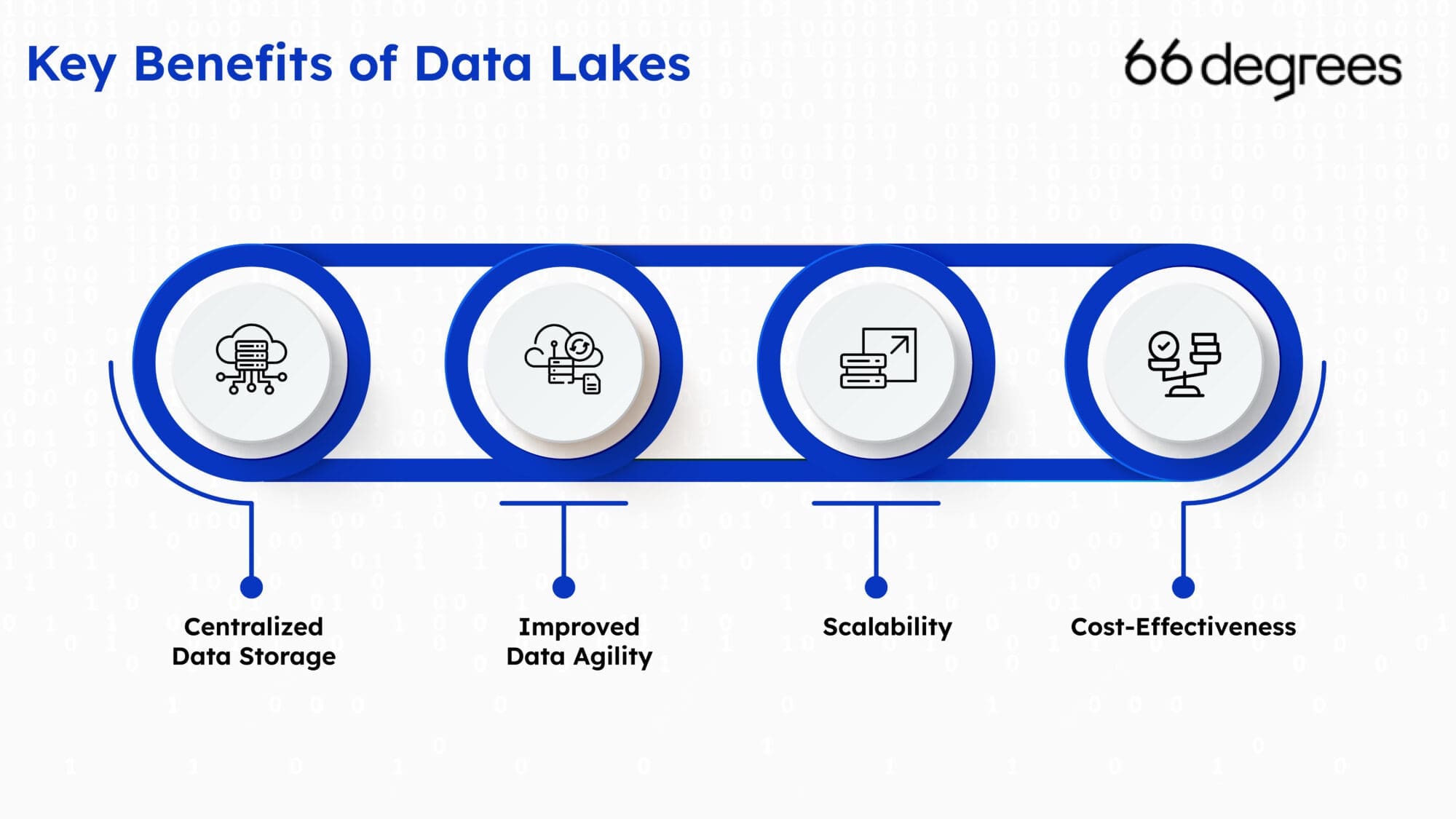
Modern data lakes play a crucial role in supporting AI and machine learning in various ways. They provide a rich, diverse dataset for training AI models, enabling real-time data processing for time-sensitive AI applications, and facilitating data exploration and discovery for new AI use cases.
Furthermore, modern data lakes support the entire AI lifecycle, from development and deployment to ongoing monitoring. Streaming data and applying AI models in near real-time enables real-time decision-making capabilities, proving invaluable in scenarios such as fraud detection, predictive maintenance, and personalized customer experiences.
Steps to Achieve Data Modernization
An effective data modernization journey requires a structured approach to ensure success. Here’s a step-by-step guide to help organizations navigate the process:
- Assessment of Current Data Infrastructure
- Conduct a comprehensive audit of existing data systems and processes
- Identify pain points, bottlenecks, and areas for improvement
- Evaluate the current state of data governance and security measures
- Developing a Modernization Roadmap
- Define clear objectives and desired outcomes for the modernization effort
- Prioritize modernization initiatives based on business impact and feasibility
- Create a phased implementation plan with specific milestones and timelines
- Implementation Strategies and Best Practices
- Choose the right cloud platform and tools (e.g., Google Cloud)
- Adopt a hybrid or multi-cloud approach if necessary
- Implement data governance frameworks from the outset
- Ensure proper data cleansing and migration procedures
- Invest in training and change management to facilitate adoption
- Measuring Success and ROI of Data Modernization Efforts
- Define key performance indicators (KPIs) aligned with business objectives
- Monitor and measure improvements in data processing speed, accuracy, and accessibility
- Track cost savings and efficiency gains resulting from modernization
- Assess the impact on AI and analytics capabilities
- Continuously refine and optimize the modernized data infrastructure
Future Trends in Data Modernization and AI
Looking ahead, several advancing technologies and trends are predicted to shape data modernization and AI in enterprise settings. These include:
- Edge Computing: The rise of edge computing will bring data processing closer to the source, enabling faster real-time analytics and AI applications, especially in IoT scenarios.
- Federated Learning: This approach allows AI models to be trained across multiple decentralized devices or servers holding local data samples, addressing privacy concerns and enabling more collaborative AI development.
- AutoML and AI-assisted Data Management: Automated machine learning tools will democratize AI development, while AI itself will play a larger role in managing and optimizing data infrastructure.
- Quantum Computing: As quantum computing matures, it promises to revolutionize data processing and AI capabilities, solving complex problems at unprecedented speeds.
- Ethical AI and Explainable AI: There will be an increased focus on developing AI systems that are transparent, explainable, and adhere to ethical guidelines.
To prepare for future data challenges and opportunities, organizations should:
- Invest in flexible, scalable data infrastructure that can adapt to emerging technologies
- Foster a culture of continuous learning and innovation within their data teams
- Stay informed about regulatory changes and evolving data privacy standards
- Collaborate with industry partners and academic institutions to stay at the forefront of data science and AI research
- Prioritize sustainability in data center operations and AI model training
66degrees: Enabling Seamless Data Modernization
As we now know, data modernization is the critical foundation for enterprise AI success. With the help of modern data infrastructure, organizations can unlock the full potential of their data assets, drive innovation, and gain a competitive edge in their industry.
Google Cloud provides a robust platform for data modernization, offering scalability, security, and cutting-edge tools enabling business owners to seamlessly accelerate AI development within their organisation.
As a premier Google Cloud partner, 66degrees specializes in guiding organizations through their data modernization journey. 66degrees offers a comprehensive suite of services to support your data modernization efforts:
- Data infrastructure assessment and strategy development
- Cloud migration and modernization planning
- Implementation of Google Cloud data solutions
- AI and machine learning integration
At 66degrees, we empower organizations to make the right choices when it comes to cloud modernization and setting the stage for AI integration. Our strategic Google Cloud consulting services help align your technology needs with your business objectives, ensuring a robust, future-proof AI infrastructure. By making use of our deep expertise in cloud, data and AI engineering, we guide you through every step of your transformation journey. Connect with us to learn how we can help.