LLM Observability: Transforming Technical Necessity into Strategic Business Asset
Diving into LLM Observability: Transforming Technical Necessity into Strategic Business Asset
Author: Stephen Witkowski | 66degrees
In high-stakes aviation, no pilot would navigate through challenging conditions without instrumentation. Yet many organizations are doing exactly that with their Large Language Model deployments—operating sophisticated AI systems without visibility into how they function in production environments.
As LLMs rapidly move from experimental projects to critical business infrastructure, this lack of comprehensive oversight creates tangible business risks. Without proper monitoring systems, organizations face potential cost overruns, degraded customer experiences, compliance violations, and brand reputation damage—all while missing opportunities to optimize performance and gain competitive advantage.
LLMs Are Transforming Business—and Creating New Risks
The LLM market is experiencing explosive growth—projected to surge from $1.59 billion in 2023 to $259.8 billion by 2030, with a staggering 80% annual growth rate. By 2025, LLMs will power approximately 750 million applications and potentially automate 50% of digital work.
Unlike traditional software, LLMs understand context, generate human-like text, and process vast amounts of unstructured data. This power introduces unique vulnerabilities:
- Hallucinations: LLMs can generate false information while presenting it as factual
- Data leakage: Processing sensitive information creates inherent disclosure risks
- Unpredictable costs: LLM deployment and operation expenses can be highly volatile
Real-world consequences are already evident. Air Canada faced legal repercussions after its AI chatbot provided inaccurate information to customers. In another case, a lawyer was sanctioned for submitting legal briefs containing fabricated case citations generated by an LLM.
The Business Case
LLM observability provides comprehensive visibility into applications, prompts, data sources, and outputs to ensure accuracy and reliability. When viewed through a business lens, observability delivers four critical values:
- Financial control and cost optimization through tracking token usage, API calls, and resource utilization
- Customer experience protection by monitoring reliability and preventing issues like slow responses or inaccurate outputs
- Governance, risk, and compliance assurance by monitoring for potential risks and preventing data leakage
- Competitive advantage through continuous improvement based on performance insights
The Maturity Model: Where Does Your Organization Stand?
Organizations leveraging LLMs can evaluate their observability capabilities through a four-level maturity model. Understanding your current position helps identify strategic improvement opportunities:
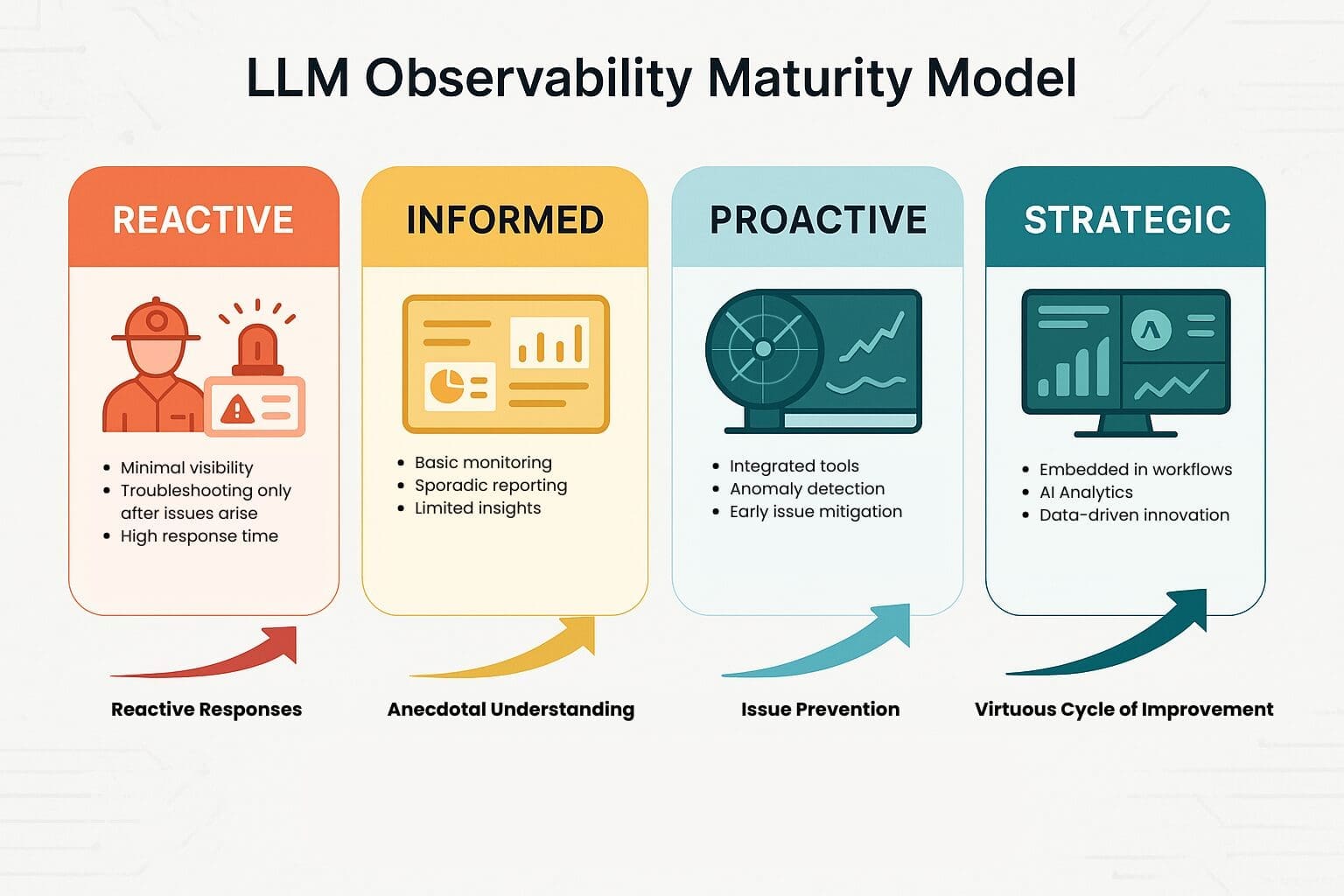
Level 1: Reactive
Organizations at this level operate with minimal visibility into their deployments, creating significant business exposure:
- Limited Monitoring Scope: Visibility restricted to basic infrastructure metricsn with little insight into specific behaviors
- Firefighting Mode: Issues only discovered after they impact users or business operations
- Manual Processes: Troubleshooting relies heavily on individual expertise rather than established processes
Siloed Information: Different teams collect disparate data with no unified view - Business Impact: Extended downtime during incidents, unpredictable costs, potential compliance violations, and customer experience degradation
When problems occur, your team struggles to determine whether issues originate from the LLM itself, surrounding infrastructure, or user inputs.
Level 2: Informed
At this stage, organizations implement foundational monitoring capabilities that provide baseline awareness:
- Structured Approach: Formal monitoring solutions with defined KPIs for LLM performance
- Centralized Visibility: Dashboards aggregating basic metrics, logs, and traces
- Historical Analysis: Capability to review past performance and identify recurring patterns
- Alert Mechanisms: Notifications for predefined thresholds and conditions
- Business Impact: Reduced troubleshooting time, improved resource allocation, and better capacity planning
Your team can identify when problems occur and has basic data to diagnose common issues, but still lacks predictive capabilities and comprehensive understanding of LLM behavior.
Level 3: Proactive
Organizations at this level implement sophisticated monitoring and analytics to anticipate issues:
- Comprehensive Coverage: Monitoring spans the entire LLM lifecycle from data ingestion to user interactions
- Anomaly Detection: AI-powered systems identify unusual patterns before they become critical problems
- Advanced Correlation: Automatic linking between metrics, logs, and traces provides contextual insights
Quality Assurance: Continuous evaluation of LLM outputs for accuracy, relevance, and bias - Business Impact: Significantly reduced downtime, optimized costs, enhanced compliance posture, and improved user satisfaction
Your team receives early warnings about potential issues, can quickly pinpoint root causes across complex systems, and has data-driven insights to optimize LLM performance.
Level 4: Strategic
At the highest level, observability becomes a key business differentiator:
- Business Alignment: Observability metrics directly tied to business KPIs and strategic objectives
- Predictive Intelligence: AI systems forecast potential issues and recommend preemptive actions
- Automated Remediation: Self-healing capabilities for common problems
- Continuous Optimization: Ongoing improvement of models, prompts, and deployment practices based on comprehensive data
- Business Impact: Maximized ROI from LLM investments, competitive advantage through superior AI performance, and resilient operations even at scale
The data actively informs executive decision-making, drives innovation, and creates measurable business value above and beyond what’s already being delivered by your AI applications.
Self-Assessment Questions
To evaluate your organization’s current maturity level, consider these three questions
- How quickly can we identify and resolve the root cause of LLM-related issues?
- Do we have real-time visibility into both technical performance and business outcomes of our deployments?
- Are we effectively optimizing costs based on usage patterns and performance data?
Building Your LLM Strategy
Implementing a robust strategy requires:
- Defining clear business goals for deployments
- Identifying key risks (cost overruns, inaccurate outputs, security breaches)
- Selecting metrics aligned with business goals and risks
Google Cloud Platform Native Observability
Google Cloud Platform offers integrated observability solutions specifically designed for monitoring cloud resources, including LLM deployments:
- Cloud Trace: Captures distributed traces across your applications, helping track request flows and identify performance bottlenecks
- Cloud Monitoring: Provides real-time visibility into performance metrics, with customizable dashboards and alerting capabilities
- Cloud Logging: Centralizes log data collection and analysis for comprehensive debugging and auditing
For organizations looking to instrument their frameworks directly, OpenLLMetry offers an open-source solution that aligns with OpenTelemetry standards. This tool enables detailed tracing of LLM operations and supports writing instrumentation data directly to Google Cloud, primarily as distributed traces in Cloud Trace.
Google Cloud Marketplace Offerings
For organizations seeking specialized LLM observability solutions, Google Cloud Marketplace offers several powerful alternatives:
- Arize AI: Provides specialized tooling for monitoring model performance, detecting data drift, and explaining LLM behaviors
- DataDog: Offers comprehensive observability with native GCP integrations and AI-specific monitoring capabilities
- Elastic: Delivers scalable monitoring, logging, and analytics with dedicated features
- Weights and Biases: Enables detailed tracking of model training and inference, with specialized tools for evaluating LLM outputs
Implementing an effective observability strategy is best approached in phases:
- Foundational Monitoring: Implement basic logging and performance metrics
- Enhanced Visibility: Add distributed tracing and output quality evaluation
- Proactive Insights: Deploy anomaly detection and predictive analytics
- Strategic Optimization: Integrate observability into development workflows
By selecting the right tools from Google Cloud’s ecosystem and establishing clear monitoring processes, organizations can transform observability from a technical requirement into a strategic business advantage.
Don’t Navigate Without a Map
Deploying LLMs without proper visibility is like navigating complex terrain without a map—exposing your organization to financial losses, compliance issues, and damaged customer trust.
Investing in it isn’t just prudent; it’s essential for future-proofing your AI investments. As these powerful models become integral to core operations, your ability to monitor performance, ensure reliability, and maintain alignment with business objectives is paramount for sustained success.
To navigate this landscape with confidence and transform a potential blindspot into a strategic advantage, schedule an assessment with the experts at 66degrees today.